There are many signals produced by the human body which carry important information of its health and emotional, state, intentions, will and several other aspects. These signals are of nonlinear and nonstationary nature, and the information is hidden and coded in a complex way which is not clearly exposed in the raw signal. Those properties degrade the performance of automatic analysis and classification systems using those signals. We use recent advanced signal processing methods like sparse representation, nonnegative matrix factorization, blind source separation, independent component analysis, regularization and inverse problems to enhance the capabilities of information extraction and improve the behavior of systems for computational analysis of that information. The objective of this research line is to produce advanced signal processing and machine learning algorithms capable of extract that information, for a wide range of applications in speech signals, in respiratory, cardiac and electroencephalography signals, among others.
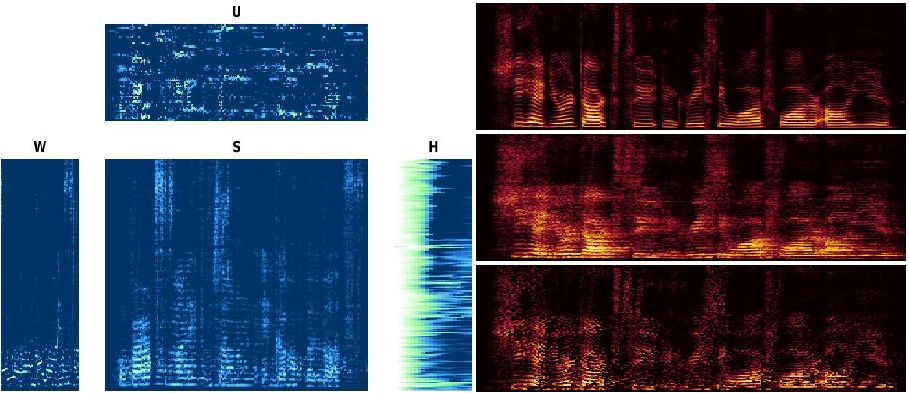
Team members
Leonardo Rufiner
Leandro Di Persia
Roman Rolon
Ivan Gareis
Jose Chelotti
Sebastian Vanrell
Web demos
fdICA: Algorithm for frequency-domain blind source separation based on the pseudoanechoic model.
multibinICA: Algorithm for frequency-domain blind source separation based on Multibin ICA for 2 by 2 mixtures.
blindder: Semi-supervised method for microRNA prediction with few labelled samples.
Beta Dereverberation: A blind, single channel dereverberation method in the time-frequency domain.
Selected publications
R. Casal, L. Di Persia, G. Schlotthauer
Biomedical Signal Processing and Control, Volume 63 – 2021
R.E. Rolon, I. E. Gareis, L. Larrateguy, L. Di Persia, R. Spies, H. L. Rufiner
Biomedical Signal Processing and Control, Volume 62, page 1–9 – 2020
R. Casal, L. Di Persia, G. Schlotthauer
Heliyon, Volume 5, Number 10, page e02529 – 2019
F. Ibarrola, R. Spies, L. Di Persia
IEEE/ACM Transactions on Audio, Speech, and Language Processing – 2019
G. Schlotthauer, A. Humeau-Heurtier, J. Escudero, H. L. Rufiner
Complexity, Volume 2018 – 2018
F. Ibarrola, L. Di Persia, R. Spies
Signal Processing, Volume 151, page 89–98 – 2018
S. R. Vanrell, D. H. Milone, H. L. Rufiner
IEEE Journal of Biomedical and Health Informatics, page 1001–1010 – 2018
R.E. Rolon, L. Larrateguy, L. Di Persia, R. Spies, H. L. Rufiner
Biomedical Signal Processing and Control, Volume 33, page 358–367 – 2017
R. Sharma, L. D. Vignolo, G. Schlotthauer, M. A. Colominas, H. L. Rufiner, S. R. M. Prasanna
Speech Communication, Volume 88, page 39–64 – 2017
F. Ibarrola, L. Di Persia, R. Spies
2017 XLIII Latin American Computer Conference (CLEI), page 1–4 – 2017
C. E. Martínez, J. Goddard, L. Di Persia, D. H. Milone, H. L. Rufiner
Digital Signal Processing, Volume 38, page 22–31 – jan 2015
S. R. Vanrell, D. H. Milone, H. L. Rufiner
IEEE Journal of Biomedical and Health Informatics, page 1001–1010 – 2018
S. R. Vanrell, J. O. Chelotti, J. Galli, H. L. Rufiner, D. H. Milone
Congreso Argentino de Agroinformática (CAI 2014) – 43º JAIIO, page 121–134 – 2014
Carlos M. Pais, H. L. Rufiner
Anales del VI Congreso Latinoamericano de Ingeniería Biomédica (CLAIB 2014), page 403 – oct 2014
D. H. Milone, L. Di Persia, G. Schlotthauer, Carlos M. Pais, L. Larrateguy
Technical Report , UNL, CONICET, Cardiocom. – sep 2013
Carlos M. Pais, H. L. Rufiner
Proc. of the 13th Argentine Symposium on Computing Technology (AST 2012 y 41 JAIIO) – 2012
C. E. Martínez, J. Goddard, L. Di Persia, D. H. Milone, H. L. Rufiner
12th Argentine Symposium on Technology (40th JAIIO) – aug 2011