Most machine learning problems deal with datasets in which every of the given examples consist of a large list of features. Usually, the set of features comes without knowledge about the discriminative information provided by each element. In this scenario, feature selection is essential to perform the classification task with reduced complexity and acceptable performance. A key issue is to define a criterion in order to rank the features, discarding those features that are less relevant, redundant, or noisy. This depends on the particular task, the classifier and the properties of the data. Another key issue comes from the infeasibility of evaluating all possible combinations, which is why an intelligent search strategy is required for finding an optimal subset. The objective of feature selection is the improvement of a machine learning model, either in terms of learning speed, computational complexity, simplicity, interpretability of the representation, and/or generalization capability.
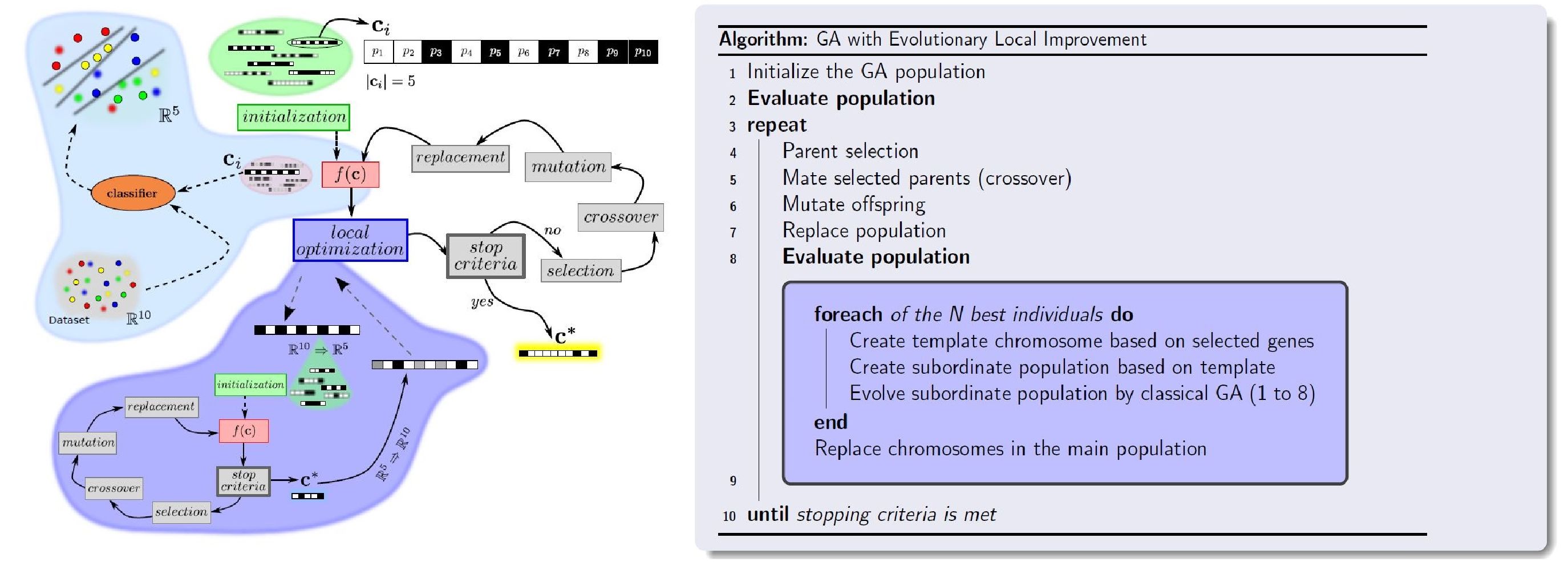
Team members
Leandro Vignolo
Matias Gerard
Publications
L. D. Vignolo, E. M. Albornoz, C. E. Martínez
AI Communications, Volume 3, Number 32, page 191–206 – 2019
L. D. Vignolo, E. M. Albornoz, C. E. Martínez
Advances in Artificial Intelligence – IBERAMIA 2018, page 455–466 – 2018
E. M. Albornoz, L. D. Vignolo, J. A. Sarquis, E. Leon
Ecological Informatics , Volume 38, page 39 – 49 – 2017
L. D. Vignolo, M. Gerard
Proceedings de XLIII CLEI – 46 JAIIO – 2017
L. D. Vignolo, S. R. M. Prasanna, S. Dandapat, H. L. Rufiner, D. H. Milone
Pattern Recognition Letters, Volume 84, page 1–7 – dec 2016
L. D. Vignolo, J. A. Sarquis, E. Leon, E. M. Albornoz
17th Argentine Symposium on Artificial Intelligence (ASAI) – 45th JAIIO – sep 2016
L. D. Vignolo, H. L. Rufiner, D. H. Milone
IET Signal Processing, Volume 10, Number 6, page 685–691 – 2016
L. D. Vignolo, H. L. Rufiner, D. H. Milone
17th Argentine Symposium on Artificial Intelligence (ASAI) – 45th JAIIO – nov 2016
L. D. Vignolo, D. H. Milone, J. Scharcanski
Expert Systems with Applications, Volume 40, Number 13, page 5077–5084 – 2013
L. D. Vignolo, D. H. Milone, J. Scharcanski, C. Behaine
2012 IEEE International Conference on Systems, Man, and Cybernetics (IEEE SMC 2012) – 2012